Generative AI: Revolutionizing Content Creation and Data Innovation
- Michael Paulyn
- Dec 12, 2024
- 4 min read
Generative AI represents a groundbreaking advancement in artificial intelligence, enabling the creation of entirely new content based on diverse inputs such as text, images, audio, and even 3D models. Unlike traditional AI models focused on classification or prediction, generative AI excels in producing original content that mimics human creativity.
With applications spanning industries from entertainment to healthcare and beyond, this technology transforms workflows, enhances data utilization, and fuels innovation. This blog examines how generative AI works, the types of models that power it, its applications, benefits, and the challenges it faces as it evolves.

Image: AI-Generated using Leonardo AI
What Is Generative AI?
Generative AI refers to using artificial intelligence to produce new, original content based on patterns and structures found in existing data. This capability leverages advanced neural networks and foundation models, such as GPT-3 for text generation and Stable Diffusion for image creation, to unlock innovative possibilities.
Unlike traditional AI, which focuses on recognizing and analyzing data, generative AI creates new data that can replicate the characteristics of the input data. For instance:
Text input can generate essays, poetry, or code.
Image input can produce new designs, 3D models, or video content.
Generative AI is particularly impactful due to its ability to work with unlabeled data through unsupervised or semi-supervised learning, making it easier to train models at scale.
How Does Generative AI Work?
Generative AI relies on neural networks and advanced learning techniques to analyze existing data and create new content. Key methodologies include:
Diffusion Models
Diffusion models operate through a two-step process:
Forward Diffusion: Adds noise to the data, gradually degrading it.
Reverse Diffusion: Reconstructs data by removing noise step by step.
This process allows diffusion models to generate highly detailed outputs, making them ideal for applications like photorealistic image generation. However, diffusion models require significant computational resources and have slower sampling speeds compared to other models.
Variational Autoencoders (VAEs)
VAEs consist of an encoder that compresses input data into a latent representation and a decoder that reconstructs the original data. While VAEs are efficient and faster than diffusion models, the quality of their outputs is often less detailed, making them suitable for applications where speed is prioritized over precision.
Generative Adversarial Networks (GANs)
GANs use two competing neural networks:
A Generator creates new data samples.
A Discriminator evaluates whether the samples are real or generated.
The iterative competition between these networks improves the quality of generated content. GANs are well-suited for domain-specific applications but struggle with generating diverse outputs.
Transformer Networks
Transformers are the backbone of many generative AI models, particularly for text-based applications. They utilize:
Self-Attention: Assigns importance to different parts of the input for contextual understanding.
Positional Encoding: Tracks the sequence of input data for coherence in outputs.
This architecture enables large language models (LLMs) like GPT-3 to generate coherent, contextually accurate text.
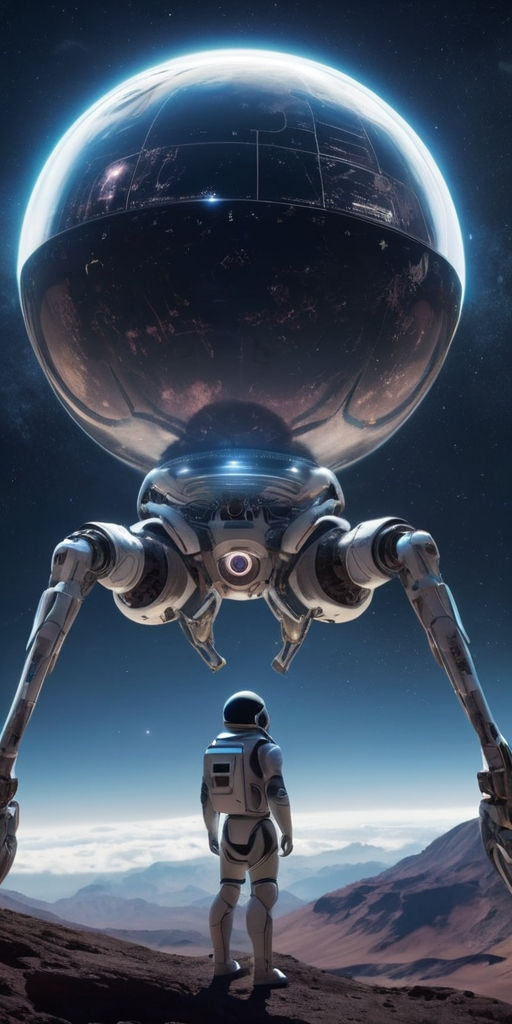
Image: AI-Generated using Leonardo AI
Applications of Generative AI
Generative AI is reshaping industries by automating complex tasks and enabling new possibilities for creativity and innovation. Common applications include:
Language
Large language models (LLMs) like ChatGPT are used for:
Essay writing
Code generation
Translation
Customer support automation
Visual Content
Generative AI powers tools for:
Image generation and editing
3D model creation for gaming and simulation
Photorealistic rendering for virtual reality and augmented reality
Audio and Music
Applications include:
Music Composition
Speech synthesis
Audio editing and enhancement
Synthetic Data
Generative models create synthetic datasets to train other AI systems, particularly in limited or sensitive data scenarios. For example:
Training autonomous vehicles using simulated driving scenarios
Enhancing medical research with artificial patient datasets
Scientific Discovery
In healthcare, generative AI aids drug discovery by modeling new protein structures. In environmental science, it helps simulate weather patterns and predict natural disasters.
Benefits of Generative AI
Generative AI offers several transformative benefits:
Creativity Boost: Automates creative tasks like writing, designing, and composing, enabling professionals to focus on innovation.
Efficiency: Speeds up content creation and data generation, saving time and resources.
Data Augmentation: Generates synthetic data to address training data gaps, improving AI model accuracy.
Versatility: Works across multiple modalities, allowing seamless transitions between text, images, and audio.
Challenges of Generative AI
While promising, generative AI faces several challenges that hinder its scalability and adoption:
Computational Complexity: Generative models require vast amounts of computational power and storage. Training these models involves processing billions of parameters and datasets, necessitating large-scale infrastructure.
Sampling Speed: Despite their high-quality outputs, Diffusion models have slow generation times. This can impact real-time applications like chatbots or interactive tools.
Data Quality and Availability: High-quality, unbiased datasets are critical for effective training. However, certain domains lack sufficient data, and obtaining commercial licenses for datasets can be costly and legally complex.
Ethical Concerns: Generative AI raises concerns about intellectual property, misinformation, and bias. Models trained on biased or limited data may produce discriminatory or inaccurate outputs.
The Future of Generative AI
Generative AI is poised to transform industries by enhancing creativity, automating workflows, and addressing complex challenges. From content creation to scientific research, its applications are expanding rapidly. However, addressing its computational, ethical, and data-related challenges will be critical for sustained growth.
This evolving technology represents the forefront of AI innovation, pushing the boundaries of what machines can create and enabling a future where AI becomes an indispensable tool for human ingenuity.
Stay Tuned for More!
If you want to learn more about the dynamic and ever-changing world of AI, well, you're in luck! stoik AI is all about examining this exciting field of study and its future potential applications. Stay tuned for more AI content coming your way. In the meantime, check out all the past blogs on the stoik AI blog!
Comments